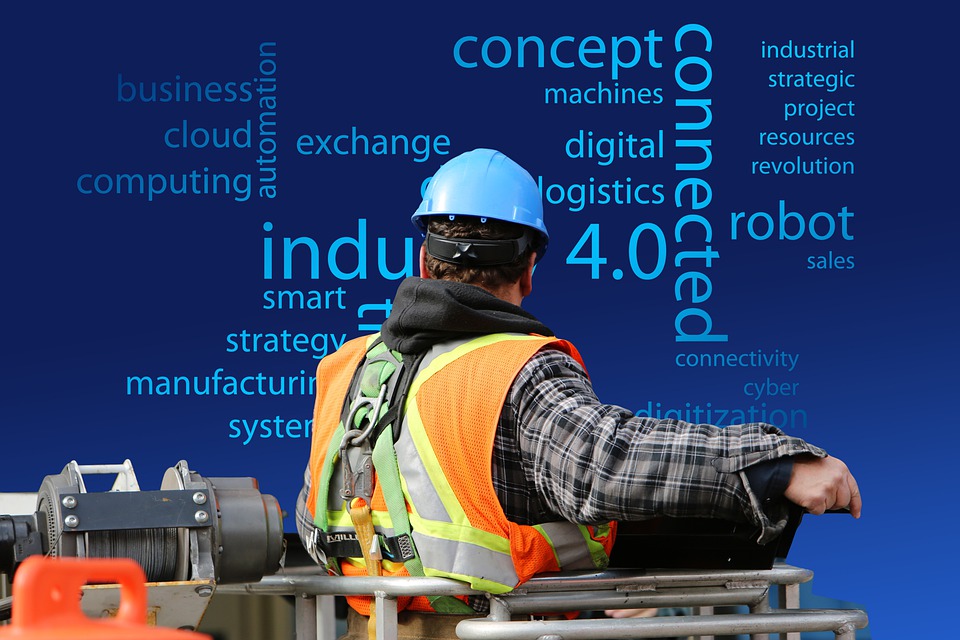
It is in the industrial sector’s best interests to tap into the potential of the large amounts of data it generates daily. Yet how can this data be used and analyzed effectively, since it is sometimes incomplete, heterogeneous or contains noise or extreme values? The DSAIDIS Chair, supported by TSN Carnot Institute and led by two research professors from Telecom Paris, seeks to meet these challenges through close cooperation with industry and service stakeholders.
The rise of big data and artificial intelligence (AI) has opened up new opportunities for businesses. They can quickly analyze large volumes of data, help with real-time decision-making and make reliable forecasts. These recent advances offer unprecedented opportunities for numerous professional sectors.
However, among these, industry and services possess certain specificities to which systems must adapt. Within these sectors, the analysis of the data collected (in considerable quantities) is fraught with challenges, including:
· Contaminated data, noise, extreme or missing values
· Conditions that change over time
· Data not or only partially labeled, i.e., with little associated information
· Heterogeneity due, among other things, to the diversity of sources used
· A sometimes insufficient amount of data, which forces the system to generate new data or operate using only a small amount.
Moreover, these obstacles occur in a context in which, for industry in particular, systems must prove that they are “trustworthy” in order to be fully embraced. The systems must therefore meet high standards demonstrating their reliability, robustness and explainability.
AI tools capable of addressing realities in the field
To meet these challenges, the DSAIDIS Chair (Data Science & Artificial Intelligence for Digitalized Industry & Services) was created with support from TSN Carnot Institute. This includes some twenty professors and researchers from Telecom Paris, who work together with the five industrial partners: Airbus Defence and Space, Engie, IDEMIA, Safran and Valeo. “We have strong ties with the companies and we each feed off each other,” says Pavlo Mozharovskyi, a research professor at Telecom Paris and co-host of the chair. “On our side, we draw inspiration from their real-life issues for our work. And our response to them provides systems that can create real added value.”
The DSAIDIS Chair aims to develop methodological tools that can be applied in realistic conditions. This approach requires a theoretical modeling phase for each project, followed by the development of algorithms, which practically apply the results previously obtained. To achieve this, researchers rely on machine learning methods, which is a branch of artificial intelligence.
“The adaptation of our systems to realistic conditions of use is central to our approach,” says Florence d’Alché, chair holder and research professor at Telecom Paris. “We do not apply our models to ideal cases, far-removed from reality. On the contrary, they are designed to adapt to noisy environments, contaminated data, extreme values, all while meeting high reliability standards and offering as many theoretical guarantees as possible.”
The tools being developed also integrate the general current issue of energy efficiency. “We make sure that our models and algorithms require as little memory, computing power and data as possible,” the researcher says. This is still a new concern within the AI community.
Four themes to increase trust in AI
The research will focus specifically on four themes established in collaboration with the five industrial partners.
1) Analysis and forecasting of time series
While this first theme may seem conventional, the researchers intend to take a new approach by combining traditional statistical methods and machine learning tools. “The original aspect of our study is our focus on a signal portion over a time interval, rather than a series of measurements at a given moment,” Florence d’Alché explains. “This approach provides additional tools to identify relevant properties to assist decision making.”
2) Large-scale mining of partially labeled and heterogeneous data
This theme has a broad scope and aims to respond to the challenges of big data in the industry and service sectors. In particular, how can we effectively mine large volumes of data, despite incomplete labeling and diverse sources?
3) Machine learning for reliable and robust decision making
The goal in this case is to strengthen user confidence in AI tools. How can machine learning algorithms take into account data imperfections (noise, contamination, extreme values), while remaining reliable? How can they help correct bias and ensure greater fairness? These issues are outside the realm of technical challenges and involve researchers in economic and social sciences.
There is also the matter of explainability. “Current machine learning models are complex and work like a ‘black box’,” Pavlo Mozharovskyi says. “We are therefore trying to explain the decisions made by the machine, so that the “black box” becomes, perhaps not white, but gray.” This wish has been strongly expressed by the Chair’s industrial partners and society in general.
4) Learning that interacts with the environment
In practice, artificial intelligence systems must be integrated into changing environments. They must therefore be able to take these developments into account and adapt their operations accordingly. The research team is therefore working to equip the tools with an autonomous and continuous learning capacity.
Fruitful collaboration between the industrial and academic worlds
The Chair has already carried out several projects in collaboration with its industrial partners. For example, Valeo turned to the researchers for help in improving one of its production lines. Like all manufacturers, the company is constantly seeking to reduce its rate of defective parts, in order to optimize performance. “We began with an extensive phase to understand the data and processes involved before analyzing them,” explains the co-host of the DSAIDIS Chair. “Significant data visualization work allowed us to identify the decisive parameters for avoiding defects during manufacturing. We then used statistical tools to make recommendations.” These were then applied to the production line with immediate results: the rate of defective parts was almost halved!
This success led to the launch of an ongoing CIFRE thesis with the same partner on another production line. The goal here is to explain anomalies that occur during manufacturing and to identify likely sources. “Our statistical analysis helps determine which jobs on the production line are most likely to cause defects in the parts,” says Pavlo Mozharovskyi. “This shows the manufacturer where to focus their efforts to improve their process.” The teams at Valeo have already started to use this tool in the field.
The researchers also worked with IDEMIA, which develops biometrics solutions that include facial recognition systems. A technology that has often been criticized for being unfair. “Our goal is to correct selection bias,” the chair holder says. “In practical terms, our system aims to either reweight learning data to reduce the effects of the under-representation of certain population groups, or to ignore certain attributes (e.g., gender, skin color).”
Through these examples, the DSAIDIS Chair perfectly illustrates the possible synergy between the industrial and academic worlds. And despite an official closure date scheduled for the end of 2023, the adventure is not expected to stop this year. “We are currently working to renew the Chair,” says Florence d’Alché. “We are meeting with each of the partners to determine which previously studied subjects should be extended and identify new issues and propose other areas of study.” This reflection has also been fueled by meeting new industrial partners, who could join the chair in 2024.