FLORIA: Detecting Floods in Urban Areas Using Satellite Imagery and AI
27 July 2023 • industry of the future
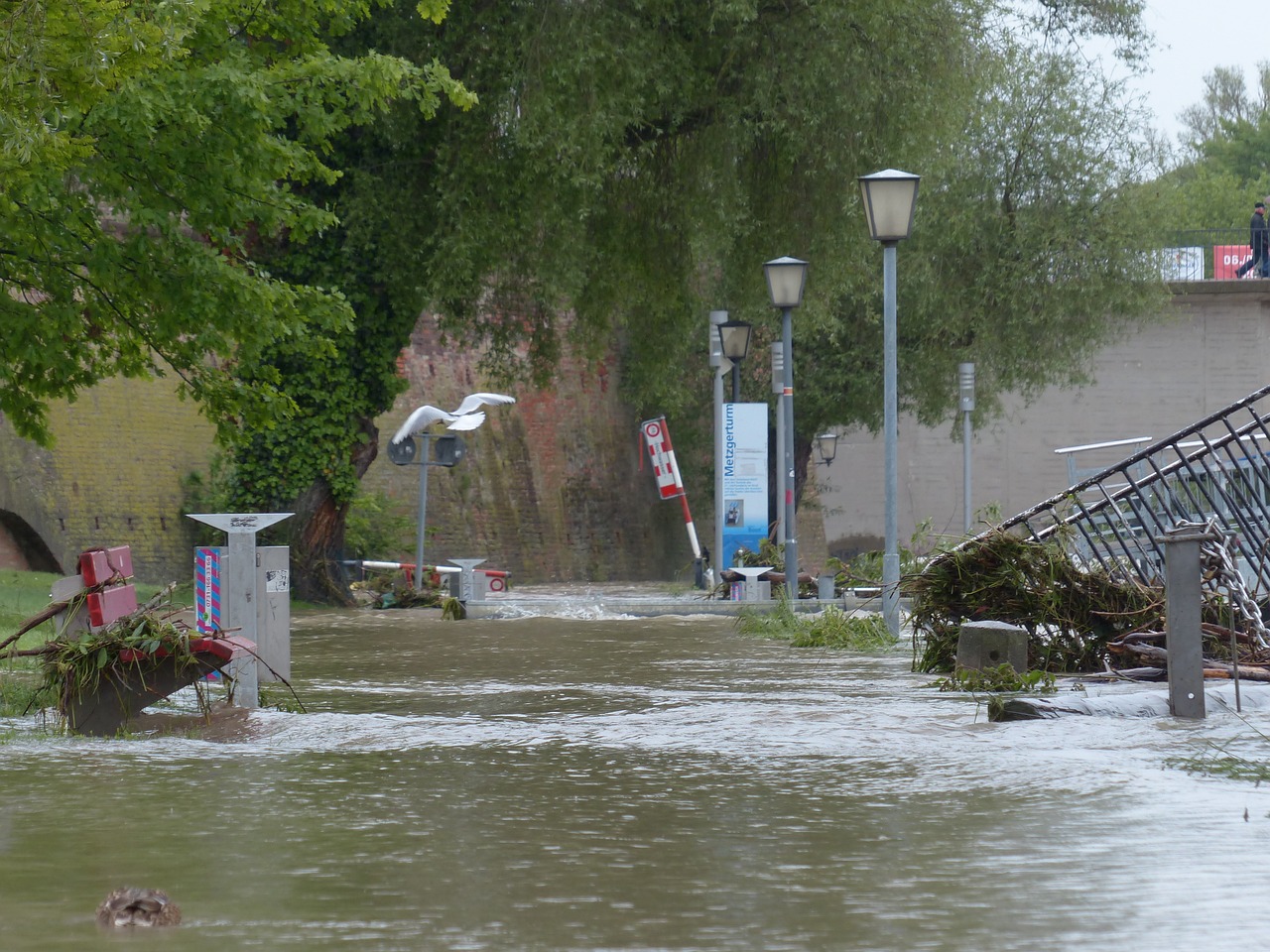
When floods strike, every minute counts. To help emergency services respond quickly, the ICube-SERTIT platform has launched the FLORIA project with support from TSN Carnot Institute to detect excess water in urban areas by analyzing satellite images using deep learning techniques.
Detecting floods as quickly as possible is a major challenge for both public authorities and the people affected. Early alerts help optimize the response of emergency services, detect roads that are passable and those that are not, assess the budget needed for rehousing, reconstruction, etc. The ICube laboratory’s SERTIT platform offers a rapid mapping service using satellite images aimed at optimizing responsiveness to natural disasters. And it can do this anywhere in the world, without relying on measurement equipment installed in the areas affected.
The FLORIA project, launched in 2022 with support from TSN Carnot Institute, was designed with this same purpose in mind. Its goal: design an automated flood detection system in urban areas, based on specific satellite data. “We use images provided by the Sentinel-1 mission, as part of the European Space Agency’s Copernicus program,” says Ari Jeannin, a radar remote sensing engineer at the ICube-SERTIT platform. “First of all because the images are available for free. But also because of the ability the mission’s satellites have to operate day and night, including in cloudy conditions.” This is significant since floods are often accompanied by thick cloud cover.
Studying the amplitude of the signal after reflection
The reason the FLORIA project focuses on urban flooding is that the analysis of satellite data in such areas is more complex than outside cities. “The onboard radar sends a wavelength towards the ground, the path of which will depend on the obstacles encountered,” the engineer explains. “In the case of urban structures, the signal will bounce off the road and then off buildings before returning to the satellite. In lowlands, on the other hand, there are only a few elements to return the signal, such as the roughness of the soil or vegetation. But in case of flooding, water behaves like a mirror on the ground reflecting the entire wavelength emitted, which will no longer return to the satellite at all.” In lowlands, therefore, a single image provides enough information to infer the presence of excessive amounts of water on the surface.
When flooding occurs in an urban environment, on the other hand, the radar’s wavelengths bounce off the water and then the buildings before eventually returning to its starting point. In this case, the difference indicating the presence or absence of flooding is less perceptible. There is therefore only one solution: comparing several images and studying each one to determine the amplitude of the signal returned to the satellite. Since water has a higher reflection coefficient than asphalt, the signal will bounce off the ground more than usual during a flood before reaching the buildings. As a result, the satellite will receive a wavelength of greater amplitude than usual.
Monitoring a loss of consistency
So, is comparing the amplitudes of several radar images all it takes? “Unfortunately, it’s not that simple,” says Ari Jeannin. “Amplitude increases can have several causes, such as atmospheric effects. This is why we combine this analysis with radar interferometry.” This technique consists in comparing the “consistency” between two images by studying a second characteristic of the radar wave: its phase, which also depends on the objects in the signal’s path. The consistency therefore reflects the degree of similarity between the phases of two satellite passages.
“In urban structures, unlike agricultural and green environments, consistency is generally high, since buildings and roads are rather stationary,” the engineer adds. “We are therefore particularly interested in areas showing a decrease in consistency between two images.” This indicator, combined with an increase in signal amplitude at the same location, is therefore a sign of probable urban flooding.
A three-image analysis
Ultimately, FLORIA requires three satellite images: two before the occurrence of the event, in order to study usual amplitude and consistency, and a third to identify any significant differences. The solution therefore remains dependent on the quantity of images provided, and the passage of the satellites over the area concerned. “However, the increase in satellite constellation projects should lead to more sources of information in coming years,” says Ari Jeannin.
The project team first developed an automated system to optimize the processing of radar data. This tool, which takes the form of a library in Python programming language, can automatically extract information such as amplitude or consistency, from the raw images provided by Sentinel-1. This library has also been encapsulated so that it can be easily transferred from one computer to another.
Forecasts powered by deep learning
This automated processing provides large amounts of data from the three satellite passes. This data must then be analyzed to infer the probability of the occurrence of a flood in an urban environment. This is a task which quickly poses difficulties for the human eye. FLORIA therefore relies on artificial intelligence, in this case deep learning techniques.
“We have benefited from recent advances in AI,” says Ari Jeannin. “We detected a lot of false positives during our first tests with conventional machine learning: areas indicated as likely to be flooded, when we knew this was impossible. This is due to the noise present on the radar images. Our deep learning algorithms have greatly reduced this error rate by taking into account the probability of a neighboring pixel being affected.” If the supposed flood appears to be restricted to a narrow and isolated area, it is likely to be inaccurate. FLORIA also takes into account the shape drawn by the pixels supposedly affected by the incident. For example, the tool will view a star-shaped flood as improbable.
FLORIA: already functional with continuous learning
However, deep learning algorithms must always complete an essential training phase. The project team therefore had to build a relevant and sufficient database from scratch. This required an extensive amount of work. “We began by listing around one hundred floods, keeping only those covered by Sentinel-1,” Ari Jeannin recalls. “We then relied on our expertise, at SERTIT, to draw up handwritten maps representing these past incidents by validating our observations using research from news sites and social media.” The engineers were then able to verify the relevance of FLORIA’s predictions by comparing them to their mapped descriptions of the floods studied.
This confirmed that the demonstrator developed was already capable of establishing relevant forecasts in urban areas. “Nevertheless, we know that our model would be more accurate if it had more training data,” Ari Jeannin says. This is one area of improvement the project team will focus on.
Even as the tool continues to prove itself, in particular through the EU CEMS-RM program, SERTIT intends to optimize the quality of the results provided. This involves regular training of the model, work on AI parameters, and the use of numerical indicators to measure the relevance of the solution and monitor its progress.
Processing time is another indicator that needs improving. “A FLORIA extraction currently takes between four and seven hours,” says Ari Jeannin. “We would like to reduce this time to a maximum of two hours, in keeping with SERTIT’s responsiveness objectives.” To do this, the team intends to export its tool to the HPC (High Performance Computing) platform at the University of Strasbourg. It hopes that its flood forecasts for urban areas will soon speed up the relief sent to the people affected.